Tim G. J. Rudner
Data Science Assistant Professor, Faculty Fellow, and Instructor, Center for Data Science, New York University
AI Fellow, Center for Security & Emerging Technology, Georgetown University
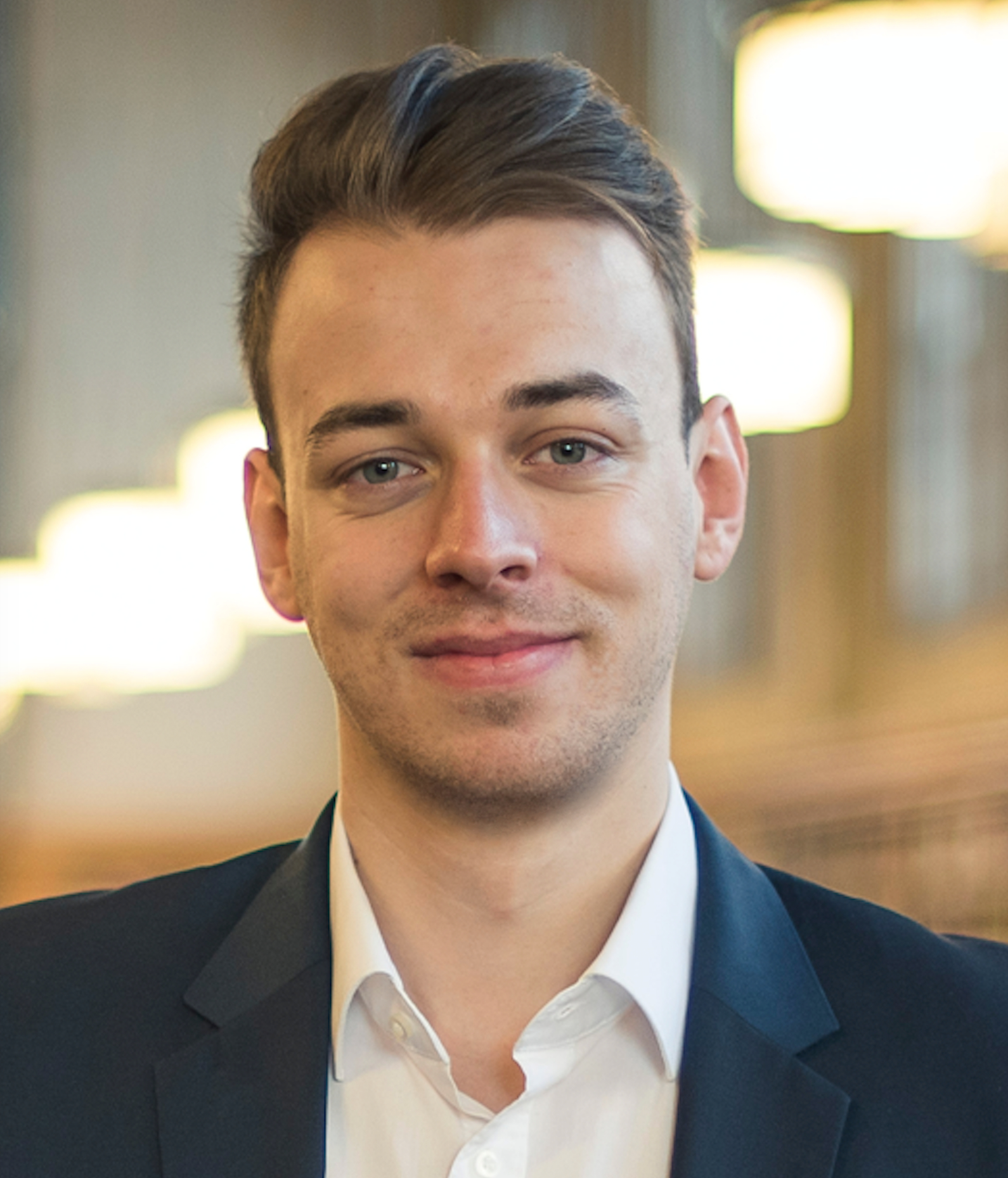
tim.rudner [at] nyu.edu
The goal of my research is to create trustworthy machine learning models with
Bio: I am a Data Science Assistant Professor, Faculty Fellow, and Instructor at New York University. Before joining New York University, I conducted PhD research on probabilistic machine learning in the Department of Computer Science at the University of Oxford, where I was advised by
I am also an AI Fellow at Georgetown’s Center for Security & Emerging Technology and a Rhodes Scholar.
Mentoring: I was the first in my family to attend college, and I know that navigating higher education can be challenging for first-generation low-income students. If you identify as a first-generation low-income student and are looking for mentorship, please feel free to get in touch using this form.
I am on the academic job market this year (2023-24). Please feel free to reach out if you think I would be a good fit for your department. You can find my CV here.
News
Apr '24 | Our work on language-guided control won an outstanding paper award at the GenAI4DM Workshop! |
---|---|
Mar '24 | I was awarded a $700,000 Foundational Research Grant to improve the trustworthiness of LLMs! |
Feb '24 | I’m leading New York University’s effort to help establish the United States AI Safety Institute! |
Dec '23 | I will be co-organizing the 6th Symposium on Advances in Approximate Bayesian Inference (AABI)! |
Dec '23 | I published four papers at NeurIPS 2023 (including a spotlight): [1,2,3,4]! |